ELTE-SMARTER Research Group
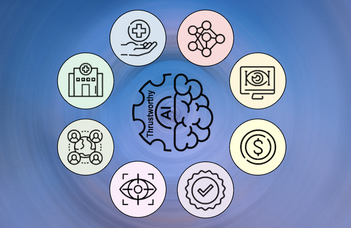
Description of Activities
The research group is engaged in model-driven machine learning, a new and rapidly evolving area of explainable artificial intelligence (XAI), which integrates mathematical and physical heuristics (e.g., sparsity, smoothness, dynamic characteristics) into the architectures, loss functions, or even the training data of machine learning algorithms. This approach helps reduce the heavy data dependency and complexity of deep learning, while making the decision-making mechanism of the trained models interpretable. The research outcomes are utilized in safety-critical applications, such as autonomous vehicle control, healthcare, intelligent transportation and energy systems, where, in addition to performance, the interpretability and transparency of decision-making are crucial.
Research Interests
- Model-driven AI: we combine parametrized orthogonal projections, convex optimization techniques, and differential equations with AI models using different model-driven AI paradigms, i.e., variable projections, deep unfolding, and physics informed learning.
- Post-hoc XAI: Model-driven AI offers transparent decision-making, but may not align with domain expertise. For example, a mathematically explainable model might extract frequencies and statistics, yet be unclear to a cardiologist who relies on ECG morphology and timing for diagnosis. Therefore, we integrate model-based AI approaches with post-hoc interpretability analysis to offer valuable insights for practitioners and machine learning end users.
- Safety-critical applications: adopting model-based XAI methods in thermographic imaging for non-destructive testing, vehicle vibration analysis for brake disc deformation and bearing fault detection, multimodal biosignal analysis, such as EEG,
- ECG, and PPG processing, traffic load estimation and near-miss situation prediction in urban mobility, and renewable energy forecasting.
- Edge hardware implementation: developing resource-efficient learning procedures that use less training data and computational power, fast algorithms for facilitating real-time processing and embedded AI solutions.
Research Methodology
- Embedding physics-based priors, such as symmetries, and conservation laws, by hierarchical design of neural networks’ (NNs) architecture like graph NNs. Introducing learning biases using dynamical equations and soft penalty constraints in the loss.
- Converting iterative numerical algorithms (e.g., ADMM, FISTA) into neural networks, where each layer mimics an iteration with trainable parameters.
- Adapting post-hoc AI research methodologies, such as visualizations (heatmaps, t-SNE), case-based reasoning, evaluating feature attribution by Shapley values and integrated gradient methods.
Research Staff
Dr. Habil. Péter Kovács, associate professor (Head of Group, kovika@inf.elte.hu), Dr. Tamás Dózsa, assistant professor (dozsatamas@sztaki.hu), 2 PhD students, and a number of BSc and MSc students are employed for conducting project subtasks.
Projects
1 national project (ELTE University Excellence Program EKA-KCS-24, Nr.: 2024/089-P253-1),
1 international project (DAAD Programmes for Project-Related Personal Exchange 2024, Nr.: DAAD-2025-26-000009), and 3 industrial projects with Siemens Mobility Hungary Ltd., Centre for Budapest Transport (BKK) Zrt., Research Center for Non-Destructive Testing GmbH.
5 important publications in the field
- T. Dózsa, C. Böck, J. Meier, P. Kovács, Weighted Hermite Variable Projection Networks for Classifying Visually Evoked Potentials, IEEE Transactions on Neural Networks and Learning Systems, 2024. DOI: 10.1109/TNNLS.2024.3475271
- E. Idrobo-Ávila, G. Bognár, D. Krefting, T. Penzel, P. Kovács, N. Spicher, Quantifying the Suitability of Biosignals Acquired During Surgery for Multimodal Analysis, IEEE Open Journal of Engineering in Medicine and Biology, 2024, DOI: 10.1109/OJEMB.2024.3379733.
- T. Dózsa, V. Jurdana, B. S. Šegota, J. Volk, J. Radó, A. Soumelidis, P. Kovács, Road Type Classification Using Time-Frequency Representations of Tire Sensor Signals, IEEE Access, 2024, DOI: 10.1109/ACCESS.2024.3382931.
- T. Dózsa, P. Őri, M. Szabari, E. Simonyi, A. Soumelidis, I. Lakatos, Brake Disc Deformation Detection Using Intuitive Feature Extraction and Machine Learning. Machines 2024, DOI: 10.3390/machines12040214.
- P. Kovács, B. Lehner, G. Thummerer, G. Mayr, P. Burgholzer, M. Huemer, Surfing Virtual Waves to Thermal Tomography: From model- to deep learning-based reconstructions, IEEE Signal Processing Magazine, 2022. DOI: 10.1109/MSP.2021.3120978
Link: https://modelinglab.inf.elte.hu/home/research-group?id=3
Elérhetőség: Dr. Habil. Péter Kovács, associate professor, kovika@inf.elte.hu