EIT Digital – Industrial PhD position proposals
EIT Digital – Industrial PhD position proposals
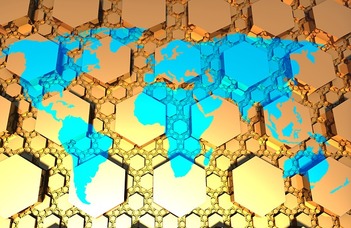
Coopetitive, multi-stakeholder resource, service and business models for digital infrastructures: Vendor Customizations over Open Networking Automation Platform (ONAP)
The thesis will model and develop strategically and financially new business opportunities for end-to-end service orchestration; proprietary extensions and upstream contribution strategies; component re-usability in alignment with the vendor’s product portfolio to achieve best market position to deliver managed distributed cloud orchestration services for Telcos, in the Open Networking Application Platform (ONAP), an open-source-based real-time, policy-driven orchestration and automation platform driven by AT&T. The Thesis will define and create multi-stakeholder workflow support for the digital network and telecommunication service ecosystem taking into account multiple operators and multiple actors, such as software vendor, virtualization lifecycle provider, OSS/BSS provider, infrastructure provider, orchestration provider and XaaS providers.
Motivation
The telco market is under transition. The Network Function Virtualization white paper was published by 13 telecom companies 13 Telcos back in 2012, that has triggered the biggest transformation of the industry to “leverage standard IT virtualization technologies to consolidate many network equipment types onto industry standards high volume servers, switches and storage, which could be located in datacentres, network nodes and end user premises” [Network Vitrualization Whitepaper, ETSI NFV (2012)]. Flexibility and on-demand service composition requires full automation of virtualization resource. Telcos & vendors must assess a new competitive landscape with software innovators and public cloud platforms entering the Telco market. Telcos’ massively distributed physical infrastructure is their key asset in competing with public cloud providers moving towards the edge. Open source initiatives – driven by Telcos – play a key role in accelerating the developments, but vendors have not built their business models on open source so far. Vendors of the future may build their business on top of and around a successful open source platform (e.g., ONAP) by adding new, substantial and differentiated extra components and sell them as a service (e.g., RedHat). The challenge is to find these extras compared to ONAP in the area of multi-stakeholder operations.
Innovation
The innovations are three-folds:
- Description and creation of an open ecosystem for new business actors and roles in the virtualization service management (e.g., Virtualization Lifecycle Management as a Service, Exchange Point Service Provider, Online Applications Service Provider, Virtualization Infrastructure Provider, Software as a Service Provider, …)
- New business models on how to operate the distributed cloud platform (a mix of open-source and proprietary extensions and managed services)
- Technical challenges related to multi-stakeholder operations, e.g., how to scale a single-point-of-customer-contact based end-to-end service delivery platform across the infrastructure of autonomous systems, 3rd party software vendors and managed services (XaaS).
This research project must take a holistic approach and seek for a coherent solution from vendors point of view including new business models, actor incentives, open source strategies, and necessary technical extensions to enable flexibly and on-demand multi-provider end-to-end service orchestration.
Expected outcome
Workload placement (Virtual Network Function (VNF) embedding) is an NP complete problem on its own but in the case of end-to-end services placement must work across multiple stakeholders. Autonomous stakeholders (service providers, brokers, vendors, …), however, want to protect their assets by exposing only minimal details necessary for cooperation with each other (e.g., AS paths in BGP routing for the Internet). Abstractions and information hiding, distributed operations, managed services, … all contribute to the increased complexity of managing distributed services. Customers, on the other hand, demands intent-based interfaces, where they can express what they want instead of explicitly defining how they want their services implemented (need secure communication for my traffic vs. buying VPN access at company sites). Thanks to virtualization, the execution environment, the product lifecycles, the configurations are continuously changing which needs high level adaptation (e.g., machine learning) from the orchestration systems. We research methods (e.g., domain abstraction models, competitive cooperation strategies, information and data models) and algorithms (e.g., workload placement, root-cause analysis, closed loop control, machine learning for model refinement) to solve the multi-stakeholder, multi-abstraction, multi-layer problem of orchestrating (design, fulfil and assure) end-to-end service at all scales: from enterprise (e.g., Industry 4.0) to end-user services. The expected academic outcomes are
- PhD dissertation
- At least one journal article with impact factor
- Several conference publications
- Industrial cooperation
Expected impact
Service lifecycle consists of service design, ervice deployment-design, instantiation, assurance and decommissioning. PhD outcome will contribute to the full automation (zero touch) of service deployment-design and a major component to closed feedback loop based service assurance by introducing standalone workload placement service component to the product architecture. Core algorithms, methods and workflows from research are channelled to this component. The first vendor to deliver such automation to service providers / enterprises will gain competitive edge and may challenge the public cloud providers. Both the Ericsson product portfolio plans and the ONAP framework envision but lack such automation component.
Location
As an industrial doctoral student, you will reside in the EIT Digital Doctoral Training Centre in Budapest and share your time with the premises of Ericsson Hungary and the Eötvös Loránd University, Faculty of Informatics. A 3-6-month mobility to another European university or research institution will be also part of the programme.
Facts
Industrial partner: Ericsson Hungary Ltd.
Academic/research partner: Eötvös Loránd University, Faculty of Informatics
Number of available PhD positions: 1
Duration: 4 years
This PhD will be funded by EIT Digital, Eötvös Loránd University, Faculty of Informatics, and Ericsson Hungary Ltd.
Apply
If you are interested in applying, please send an e-mail to Zoltán Istenes (zoltan.istenes@eitdigital.eu) including a CV, a motivation letter, and documents showing your academic track records.
Please apply before 25 July, 2019.
Customer profiling-based personalization in financial systems
Customer profiling-based personalization in financial systems
OTP Bank Hungary aims to create a model based operation and decision making environment via being among the first participants in the retail banking sector who implement advanced machine and deep learning techniques in the whole spectrum of their daily operation. The automation of predictive modeling and customer segmentation will be the cornerstone of providing real time, actionable insights for the decision makers and suitable recommendations for the clients.
Challenge
The technological revolution of the past decades caused a high speed accumulation of business related data in the retail banking sector; this trend is expected to accelerate during the coming years due to the spreading of smart appliances and online banking. Most importantly, clients’ behaviour is now recorded in more detail than ever before, with thousands of features about their demographic background, savings, investment portfolio, payment, browsing and purchasing history, and the financial products they own. The massive volume of client data – combined with external data sources like satellite images, maps, macroeconomic indices etc. – requires new analytic approaches to create useful insights for decision makers and develop/implement automated tools to collect, clean, validate, analyse data and generate real time recommendations. New methods are constantly developed in the data science community but they are mostly focused on engineering problems like image and speech recognition. The challenge is how to use these techniques for retail banking purposes, i.e. which methods and approaches have a value added in the financial sector.
Approach
In this doctoral work the state-of-the-art will be advanced in the customer profiling domain, allowing larger banks to create constantly evolving customer micro-profiles, which will allow them to provide personalized services, thereby optimizing their interactions with customers, offering the next best product and avoiding churn.
The research questions of the PhD will be formulated in line with the bank’s need to identify clients’ future behavior, which is a true pain point, whose solution has the potential to revolutionize the interaction between the bank and its customers. A draft list of novel research questions which will be analyzed during the PhD project follows: churn prediction/survival analysis, recommendation systems, data visualization.
Expected outcome
The result of the PhD will be a toolbox with implemented algorithms able to automatically collect, structure and clean customer data, give model-based recommendations and predictions. It will be implemented in a financial system’s production environment (i.e. OTP Bank) as a business supporting tool. The measurable outputs shall include documentation of existing industrial solutions in the field of research, implementation of existing solutions utilized as baselines, implemented behaviour prediction toolbox, a detailed analysis of the candidate’s contribution and the impact on the bank’s operational and business processes, published papers in journals and conferences.
Location
As an industrial doctoral student, you will reside in the EIT Digital Doctoral Training Centre in Budapest and share your time with the premises of OTP Bank Hungary and the ELTE Eötvös Loránd University. A 6-month mobility to another European university or research institution will be also part of the programme.
Facts
* Industrial partner: OTP Bank Hungary
* Academic/research partner: ELTE Eötvös Loránd University, Budapest, Hungary
* Number of available PhD positions: 1
* Duration: 4 years
* This PhD will be funded by EIT Digital, ELTE Eötvös Loránd University, and OTP Bank Hungary
Application
If you are interested in applying for this position, please send an e-mail to the EIT Digital Budapest DTC lead zoltan.istenes@eitdigital.eu, including a CV, a professional motivation letter, and documents showing your academic track records, publications, before 2019/07/25.
For further information please see the attached file.
Document library
Title | File name | |
---|---|---|
Title | File name | |
Optimization of internal processes in financial systems | IDSL-Proposal-OTP-ELTE-Lendak-Processes-accepted-f.pdf | |
Cryptographically secure, on-chain pseudorandom number generation (PRNG) | IDSL-proposal-E-Group-ELTE-Burcsi-random-accepted-f.pdf | |
Blockchain oracles | IDSL-proposal-E-Group-ELTE-Ligeti-oracleR-accepted-f.pdf | |
Towards a comprehensive Quality of Service framework for next generation networks | IDSL-proposal-Ericsson-ELTE-Laki-QoS-accepted-f.pdf | |
Customer profiling-based personalization in financial systems | IDSL-Proposal-OTP-ELTE-Lendak-Profiles-accepted-f.pdf | |
Information fusion of video analysis in ADAS system | IDSL-proposal-Bosch-ELTE-Lorincz-fusionR-accepted-f.pdf |
Anonymity and Authentication in Large Databases
Anonymity and Authentication in Large Databases
Big data has become a research hotspot in both academic and industrial areas. It is a revolution of transforming people’s ways of thinking, habits of working even the structure of society. However, big data is facing with many risks in the processes of collection, storage and use. Among of all these risks, it is obvious that the privacy and information leakage is one of the most serious issues.
The basic research directions will be anonymous data technology, identification authentication based on big data and data authenticity analysis. The main cryptographic tools to apply will be secret sharing and attribute-based cryptography.
MOTIVATION
Due to the informatization of society, data shows an explosive growth. According to statistics, there is an average of 2 million users use google search every second, more than 2 billion posts are shared by Facebook, and more than 350 million Twitters are processed every day. At the same time, IoT (the Internet of Things) makes a variety of deception and induction equipment generate data constantly. At present, after could computing, big data has become another growth point in the field of information technology. Data is becoming increasingly big but not only vertically but horizontally as well i.e. variety. Because data is big – the importance of proper data governance is sky rocketing.
Security and privacy is regarded as one of the most serious issues. All of our behaviours are controlled by network service providers. For example, Amazon knows our spending habits, google knows our search habits while Facebook knows everything about our contact information with our friends. On the other health care data is even more critical by its true nature e.g. OMICS (e.g. genomics data, proteomics data etc.) data is determining for multiple generations of an individual how past and future is or will be interpreted related to anything. Genomics combined with other healthcare data reveals hidden wisdom about our individual future, risks and potential health-opportunities. All of these practical cases show that after large quantities of harmless data are collected, it will reveal personal privacy, and the future is a complex balancing act between usability-flexibility-practicality and privacy, short term gain vs long term loss, adverse effects of lousy data governance/losing control or ability re-gain control.
Big data also faces security risks in the process of storage, processing and transmission. Service providers are both producers of data and management of data. Therefore, it is extremely difficult to restrict by technical means.
INNOVATION
In order to improve the security of big data, the following cryptographic tools will be used for the future work as two main building blocks in secure distributed system:
1. Secret sharing: it is a cryptographic method for distributing a secret to multi-parties, each of whom only takes a part of the secret. With this technique, we can build a secure, privacy-preserving system during data sharing and computation. It can solve the problem of ensuring the data is secured in sharing and computation. An interesting special case is the k-threshold case, which can be useful in k-anonymity. During the research all the GDPR-aspects must be taken into account (see Regulation (EU) 2016/679).
2. Attribute-based cryptography: it is an encryption method in which the private key is used to decrypt data dependent on users’ attributes. There are mainly two types of encryption: key-policy attribute-based and ciphertext-policy attribute-based. It avoids the disadvantage of traditional public key encryption of bonding the identity with public key. This cryptographic technology provides a much more flexible way to encrypt and decrypt. This novel tool is a possible solution for sophisticated authentication models, like behaviour or environment based authentication. Both attribute-based encryption (for selective attribute queries) and attribute-based signature may be interesting. For the latter one it is recommended to analyse operation and cryptographic techniques of IRMA-card (see I Reveal My Attributes), which uses attribute-based signatures).
EXPECTED IMPACT
E-Group expects a strong day-to-day collaboration which is enabling E-Group to access the latest research trends and applicable results to new, cutting edge software components that can fit into our main business lines, both in digital identity/attribute and digital transactions and Datalake related data sharing and digital consenting (to access personal data) problematics, especially in highly sensitive healthcare data, financial or e-government related use cases. E-Group hopes that the theoretical PhD work with practical edge to it, collaborating with E-Group experts and building into the PhD industry end-user influence from our customers’ demands will enable the candidate and E-Group to implement and utilise “low hanging fruits” from the theoretical work, ideally bringing new products or services to the market. Equally important that our Industry PhD candidate has a secondary role too: namely to catalyse our experts and specialist with new knowledge and latest scientific results that are not only new but realistically applicable to unsolved yet very important problems.
LOCATION
As an industrial doctoral student, you will reside in the EIT Digital Doctoral Training Centre in Budapest and share your time with the premises of E-Group ICT Budapest and the Eötvös Loránd University, Faculty of Informatics. A 3-6-month mobility to another European university or research institution will be also part of the programme.
FACTS
Industrial partner: E-Group ICT, Budapest
Academic/research partner: Eötvös Loránd University, Faculty of Informatics
Number of available PhD positions: 1
Duration: 4 years
This PhD will be funded by EIT Digital, Eötvös Loránd University, Faculty of Informatics, and E-Group ICT Budapest
APPLY
If you are interested in applying, please send an e-mail to Zoltán Istenes (zoltan.istenes@eitdigital.eu) including a CV, a motivation letter, and documents showing your academic track records.
Please apply before 20 October 2019.
Interviews will be scheduled during the week of 21-25 October 2019.