Machine Learning for Software Engineering Research Group
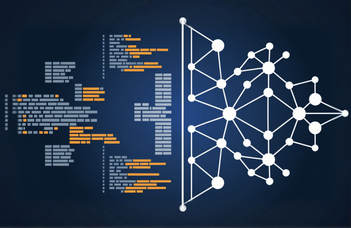
Description of Activities
The core activities concern the application of machine learning, particularly deep learning, to software engineering: teaching machines to understand, write, and fix code. This relies on the naturalness of source code that makes it possible to apply the vast knowledge accumulated in the natural processing community to computer programs.
Research Interests
- program code transformations
- code idiomatization (Szalontai et al., 2024, Szalontai et al., 2023)
- automatic bugfixing (Szalontai et al., 2024)
- neural decompilation (Szalay et al., 2024)
- requirements engineering
Research Methodology
- Recurrent Neural Networks
- Transformers
- Large Language Models
- Copying Mechanism
Research Staff
Tibor Gregorics, Associate Professor (gt@inf.elte.hu); Balázs Pintér, Senior Lecturer, 3 PhD students and more than 10 MSc and BSc Students
5 important publications in the field
- Szalay, G., Poór, M. B., Pintér, B., & Gregorics, T. (2024). Single-pass end-to-end neural decompilation using copying mechanism. Neural Computing and Applications, https://doi.org/10.1007/s00521-024-10735-9
- Szalontai, B., Vadász, A., Márton, T., Pintér, B., & Gregorics, T. (2024). Fine-Tuning CodeLlama to Fix Bugs. In Z. Illés, C. Verma, P. J. S. Goncalves, & P. K. Singh (Eds.), Proceedings of International Conference on Recent Innovations in Computing (pp. 497 509). Springer Nature. https://doi.org/10.1007/978-981-97-3442-9_34
- Szalontai, B., Márton, T., Kukucska, Á., Pintér, B., & Gregorics, T. (2024). Idiomatizing Python Source Code Using Different Recurrent Architectures. In K. Arai (Ed.), Intelligent Systems and Applications (pp. 228–242). Springer Nature Switzerland. https://doi.org/10.1007/978-3-031-66431-1_15
- Szalontai, B., Kukucska, Á., Vadász, A., Pintér, B., & Gregorics, T. (2023). Localizing and Idiomatizing Nonidiomatic Python Code with Deep Learning. In K. Arai (Ed.), Intelligent Computing (pp. 683 702). Springer Nature Switzerland. https://doi.org/10.1007/978-3-031-37963-5_47
- Mucsányi, B., Gyarmathy, B., Czapp, Á. and Pintér, B. (2022) ’Flexible Example-Based Program Synthesis on Tree-Structured Function Compositions’, SN Computer Science, 3, pp. 218:1–218:15. DOI: 10.1007/s42979-022-01090-4