Hojjat Adeli Award for Outstanding Contributions in Neural Systems
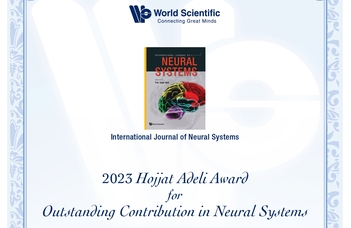
The International Journal of Neural Systems (IJNS) is a monthly rigorously peer-reviewed transdisciplinary journal (D1, impact factor: 8.0) which covers information processing in natural and artificial neural systems. Subjects of special interests are machine learning, computational neuroscience and neurology, and innovative and high-impact articles that cross multiple fields including neurosciences and computer science and engineering.
To honor the extraordinary leadership and impactful and inventive research contributions of the Editor-in-Chief of IJNS, the World Scientific Publishing Ltd. established the Hojjat Adeli Award for Outstanding Contributions in Neural Systems in 2010 awarded annually to the author(s) of the most innovative paper published in the previous volume year of the IJNS. In 2023, the prize was awarded partly to the researchers, Dr. Péter Kovács and Dr. Bognár Gergő of the Signals and Systems Research Group at the Department of Numerical Analysis of the Faculty of Informatics for the following paper:
P. Kovács, G. Bognár, C. Huber, and M. Huemer, VPNET: Variable Projection Networks, International Journal of Neural Systems, 32:1, 2150054 (19 pages).
The research was implemented in collaboration with ELTE IK, the Signal Processing Institute of the Johannes Kepler University in Linz and the Embedded AI department of the Silicon Austria Labs. The paper was published as an open access publication with supplementary material that includes the experimental data, as well as the NumPy and PyTorch implementations of the proposed method.
In this publication, we proposed a new model-driven neural network architecture that integrates parameterized orthogonal transformations. Model-driven neural network design is a recent topic in the field of Explainable AI (XAI) that combines mathematical model-based approaches with data-driven algorithms of artificial intelligence. In the above publication, numerical methods for non-linear least-squares fitting problems, which are often encountered in physics and engineering, are embedded in fully connected neural networks. The resulting VPNet is a model-driven neural network that includes the numerical and mathematical heuristics needed to solve the target problem. The model-driven network design may improve the generalization ability, interpretability, and data-efficiency of state-of-the-art deep learning models.
Since the publication of the basic concept, we have evaluated the effectiveness of VPNet in various applications related to interdisciplinary informatics, such as ECG-based arrhythmia detection, color classification of visually evoked potentials using EEG data, and the detection of potholes through tire sensor signals. Within the framework of the Modeling R+D Lab, we successfully involved several MSc students in exploring the potential practical applications of VPNet, resulting in diploma and TDK theses. We warmly welcome any interested students to participate in these ongoing research projects this year as well.